.png)
AI Doesn’t Start with Action. It Starts with Awareness
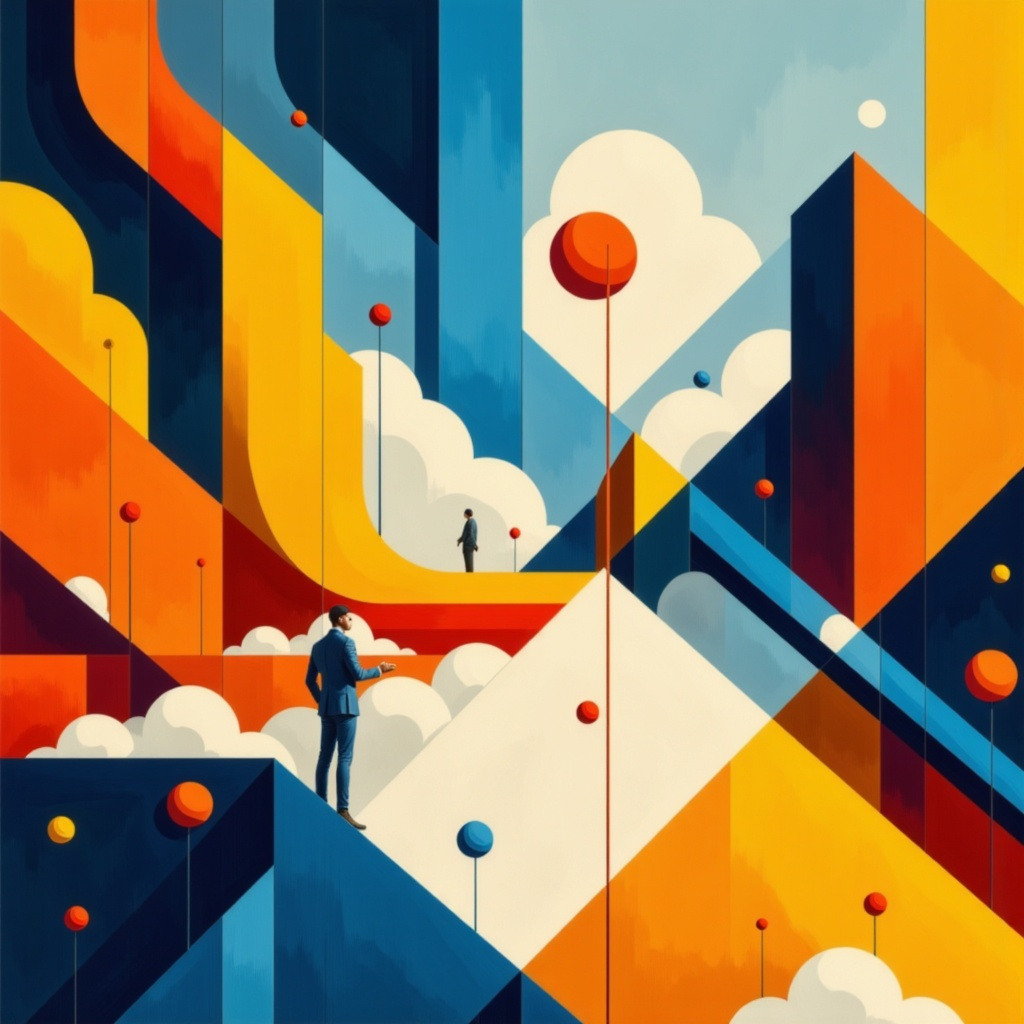
We’re in the middle of an AI gold rush. Companies are releasing new tools and features and have endless pitch decks, all promising companies that they can increase efficiency and help scale production. But here’s what doesn’t get talked about enough: the companies that are making real progress with AI aren’t the ones chasing trends. They’re the ones asking the right questions.
They’re focused on the messy, unglamorous parts of their operations. They’re identifying pain points with surgical precision. They know where they’re stuck and they’re honest about it.
Why Strategy Comes Before AI Planning
Before you spin up a pilot project or plug in another SaaS integration, pause. The best AI strategies don’t start with implementation and quick fixes but rather observation. Take time to ask yourself where your friction points are in your business. What repetitive tasks are still stealing time from your employees and energy from your teams? Where do things break down in the production or process?
It sounds simple, but most companies skip this step. They leap into action before understanding what’s actually worth fixing. And that’s where things go sideways. You end up solving problems that don’t really matter, or worse, accelerating inefficiencies.
Pain points vary wildly from business to business. A fast-scaling SaaS startup isn’t going to have the same challenges as a global logistics company. There’s no universal playbook. Context is everything. And McKinsey backs this up. Their 2023 report found that organizations with a clearly defined AI strategy are far more likely to see real, measurable results. Without a strong foundation, even the best tools will underdeliver. (McKinsey & Company, 2023)
Strategy vs. Planning: Know the Difference
Let’s be clear: planning is tactical. Timelines. Resources. Roadmaps. But strategy? That’s directional. It answers the bigger questions such as why are we doing this? What outcomes actually matter? What are we trying to change?
Planning without strategy is like setting sail with a detailed itinerary and no map. You might move fast, but there’s no guarantee you’ll end up anywhere worth going. Strategic clarity ensures every decision you make, from tools to training, actually serves a higher purpose.
A study in ScienceDirect puts it bluntly: when AI initiatives don’t align with strategic goals, they usually fizzle. They become side projects. They lose sponsorship. And eventually, they get quietly shelved. (ScienceDirect, 2023)
Tailoring AI to What Matters Most
The flashiest AI tool isn’t always the right one. What matters is whether it’s helping you solve something real.
KPMG understood this when they built AVA, their internal virtual assistant. They didn’t start with tech but with a list of pain points: manual tasks, duplicated data entry, time lost to inefficiency. AVA was designed to fix those problems. It worked. Not because it was innovative, but because it was specific. (techUK, 2024)
This is the real value of tailoring AI. You’re not building for some hypothetical future state. You’re solving today’s real frustrations. You’re streamlining processes people actually use. That’s what makes AI stick. That’s what turns it from hype into impact.
Real-World Examples: Where AI Meets Business Outcomes
Looking for a few examples of companies getting right? Here are two that took it to whole other level. What unites both cases? Clarity. They knew exactly what they wanted to fix before they brought in AI.
Deloitte’s Zora AI
Deloitte focused on their finance functio, an area bogged down by repetitive, manual work. They took time to build Zora AI to free up time and reduce overhead. Result? A 25% cost reduction and a 40% boost in productivity.
Zora didn’t succeed because it was the most advanced AI on the market. It succeeded because it was built to do something useful. Deloitte knew the outcome they wanted: fewer hours wasted, more time on high-value work. And they designed the solution backwards from that.
EY’s Agentic Platform
EY rolled out an AI agent system across their tax division. Why? Because regulatory compliance and research tasks were eating up valuable hours. Their solution? 150+ AI agents now handle the heavy lifting. The platform works because it’s laser-focused on a known, high-impact issue.
They framed their AI as a way to modernize a business-critical function. That shift in mindset made all the difference.
Leadership’s Role: Ask Better Questions
You don’t need to be a technologist to lead in this space. What you need is curiosity and clarity. The best leaders don’t ask, “How do we use AI?” They ask, “What problems are worth solving?”
That question changes the conversation. It sets the tone for how teams approach the tech—and it puts the focus where it belongs: on outcomes, not optics. It invites people to challenge outdated processes and think critically about what’s working and what’s not.
This shift in questioning aligns closely with what Harvard Business Review refers to as the “AI Leadership Matrix.” The most effective leaders aren’t just operationally excellent—they’re strategically curious. They know how to balance human insight with machine intelligence. And they know that no algorithm can replace the human responsibility of asking why. (Harvard Business Review, 2024)
Don’t Automate the Wrong Things Faster
This might be the biggest pitfall in AI adoption: using it to scale something already broken. If your process is inefficient, AI won’t fix it—it’ll just make it faster. If your data is messy, AI will amplify the noise. And if your systems aren’t aligned, AI won’t magically connect the dots.
It’s a tough truth but one worth repeating: AI is a multiplier, not a miracle. It can’t solve structural issues. BUT it can’t rethink your workflow.
Working out the job of AI is your job. But once you’ve done the work, it can scale what’s already good.
Before you implement, ask: “Is this worth scaling?” If the answer’s no, fix the process first. Then bring in AI to accelerate what’s already working.
What AI Can—and Can’t—Do Well
Knowing what problems AI is good at solving is just as important as knowing your business priorities. Not every challenge is a good match for automation or prediction. Successful AI initiatives start with realistic expectations about the kinds of tasks AI can support.
AI Works Well For |
AI Struggles With |
Repetitive, rules-based tasks |
Complex judgment calls |
Pattern recognition in large data sets |
Abstract reasoning or empathy |
Forecasting based on historical trends |
Strategy-setting and vision |
Document classification and extraction |
Managing culture and behavior |
Customer segmentation and targeting |
Ethics-based decision-making |
Use this as a reality check before diving into any initiative. If the problem lives in the right-hand column, AI might support parts of the process but it won’t replace your leadership, judgment, or intuition.
Focus Before You Scale
It’s easy to be impressed by what AI can do. But the companies pulling ahead are clear on where they are headed, what needs to change, and why AI is the right fit for that journey.
That clarity? It’s your unfair advantage. Strategy-first companies move with intention. They’re not overwhelmed by the noise. They know their problems. They know their value drivers. And they build AI around that—not the other way around.
Already on this path? Let’s swap notes. I’d love to hear what you’re learning.